As any TikTokker, Zoom presenter, or amateur photographer worth their salt can tell you, sometimes a good background is all you need to make your content really pop. It turns out that the same principle applies when trying to figure out which promoter DNA hypermethylation events drive tumor growth. MethSig, a new algorithm developed by Heng Pan in Dan Landau’s group at Weill Cornell Medicine, improves the identification of cancer-driving DNA methylation events by factoring in local background methylation rates.
But first, some background — and remember, it’s all about the background. Establishing which instances of hypermethylation contribute to cancer growth and which are just “passenger” events stochastically emerging alongside these “driver” events is easier said than done. Certain genes might be generally more prone to hypermethylation than others, and altered methylation is itself a feature of certain cancers, including chronic lymphocytic leukemia (CLL).
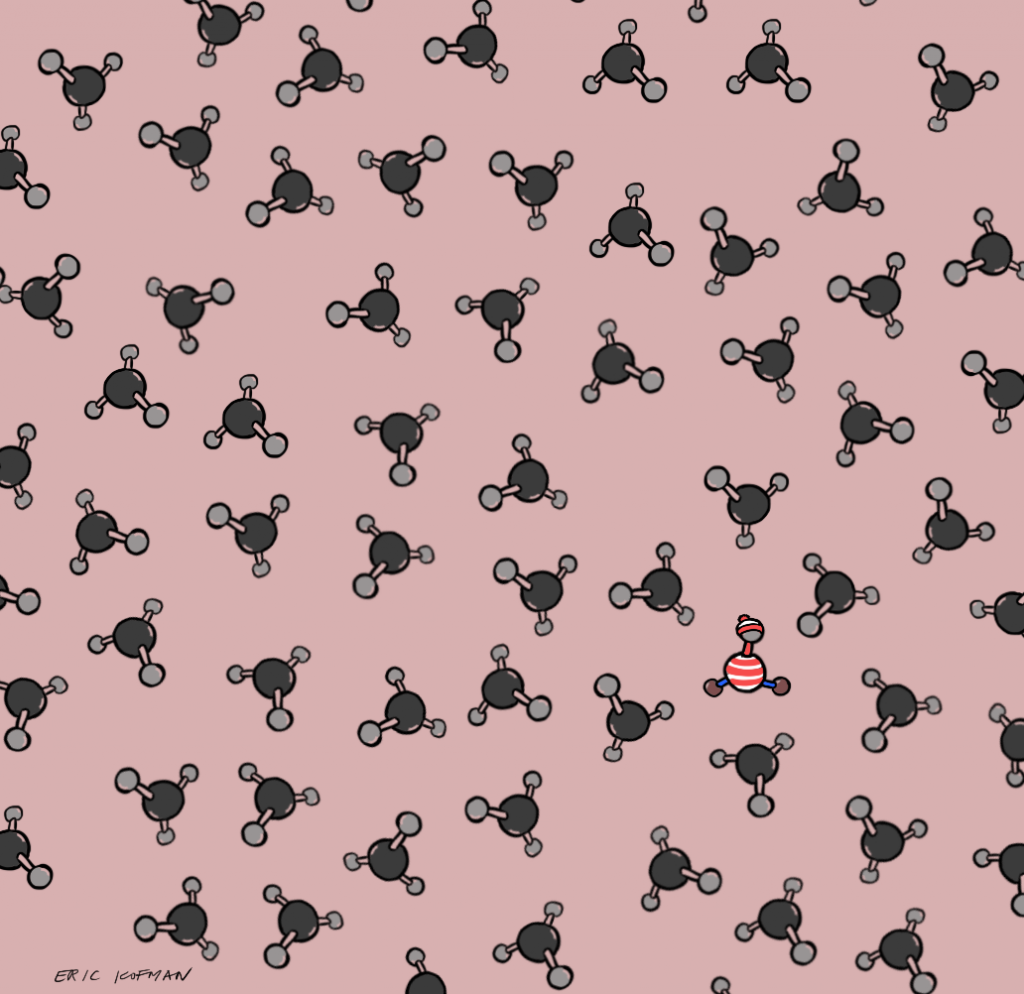
Luckily, even seemingly random methylation alterations have a gene-specific signature influenced by gene expression and replication timing. By incorporating these covariates as well as local background methylation rates and CpG within-read concordance into its underlying beta regression model, MethSig improves on existing epigenomics methods that tend to assume a uniform background methylation rate, a less nuanced approach that can result in a higher false positive rate in identifying driver methylation events.
MethSig was initially trained and tested on reduced representation bisulfite sequencing (RRBS) data from the previously published CLL8 cohort, and validated on a separate cohort of previously published data from the Dana Farber Cancer Institute (CLL-DFCI). Here’s the nitty-gritty on the validation approaches used to establish MethSig as a game-changer when it comes to studying oncogenic promoter hypermethylation:
- MethSig yields better-calibrated (less inflated) Q-Q plots than all benchmarked methods, across various tumor types including multiple myeloma and ductal carcinoma in situ
- Yes, this means fewer significant p-values and hence fewer candidate promoters, but this gives us higher confidence in the ones it does identify
- MethSig nominates a larger set of overlapping driver genes across two CLL datasets than previous methods, pointing to greater statistical robustness in its approach
- MethSig candidates are more highly enriched for genes undergoing silencing, as determined by RNA expression levels in the same samples
- Pathway enrichment analysis on candidates MethSig identifies in various cancers yields enrichment for genes regulated by Myc or p53, both of which are known to be silenced in cancers
- MethSig-array, a variant of MethSig uniquely adapted to work with Infinium HumanMethylation450 arrays from the TCGA Pan-Cancer analysis project, is able to predict both gene silencing and clinical outcome better than benchmarked methods
Of course, if you wanted to trust algorithms blindly you could just while away your time on TikTok. Landau’s team functionally verified their algorithm’s predictions by checking that the novel hypermethylation targets it nominated, such as RPRM and SASH1, do indeed confer increased resistance to CLL treatment ibrutinib in CLL-derived cell lines, using a CRISPR/Cas9 knockout system to mimic the effects of silencing these candidate genes.
Summarizing MethSig’s potential prognostic utility, first author Heng Pan shares, “The classifier we developed using MethSig produced estimated risks for each patient, and we found that patients with higher estimated risks were more likely to have had worse outcome.” Senior author Dan Landau concludes, “Ultimately we envision being able to map the entire landscape of cancer-driving DNA methylation changes, for different tumor types and in the contexts of different treatments, so that we can expand the scope of precision medicine beyond genetics to include also the critical dimension of epigenetic changes in cancer.”
Learn how to cut through the background noise of cancer methylomes in Cancer Discovery, May 2021.